Pleanary talks will be given in the main conference hall and have duration 50′ for the talk and 10′ for questions and discussion.
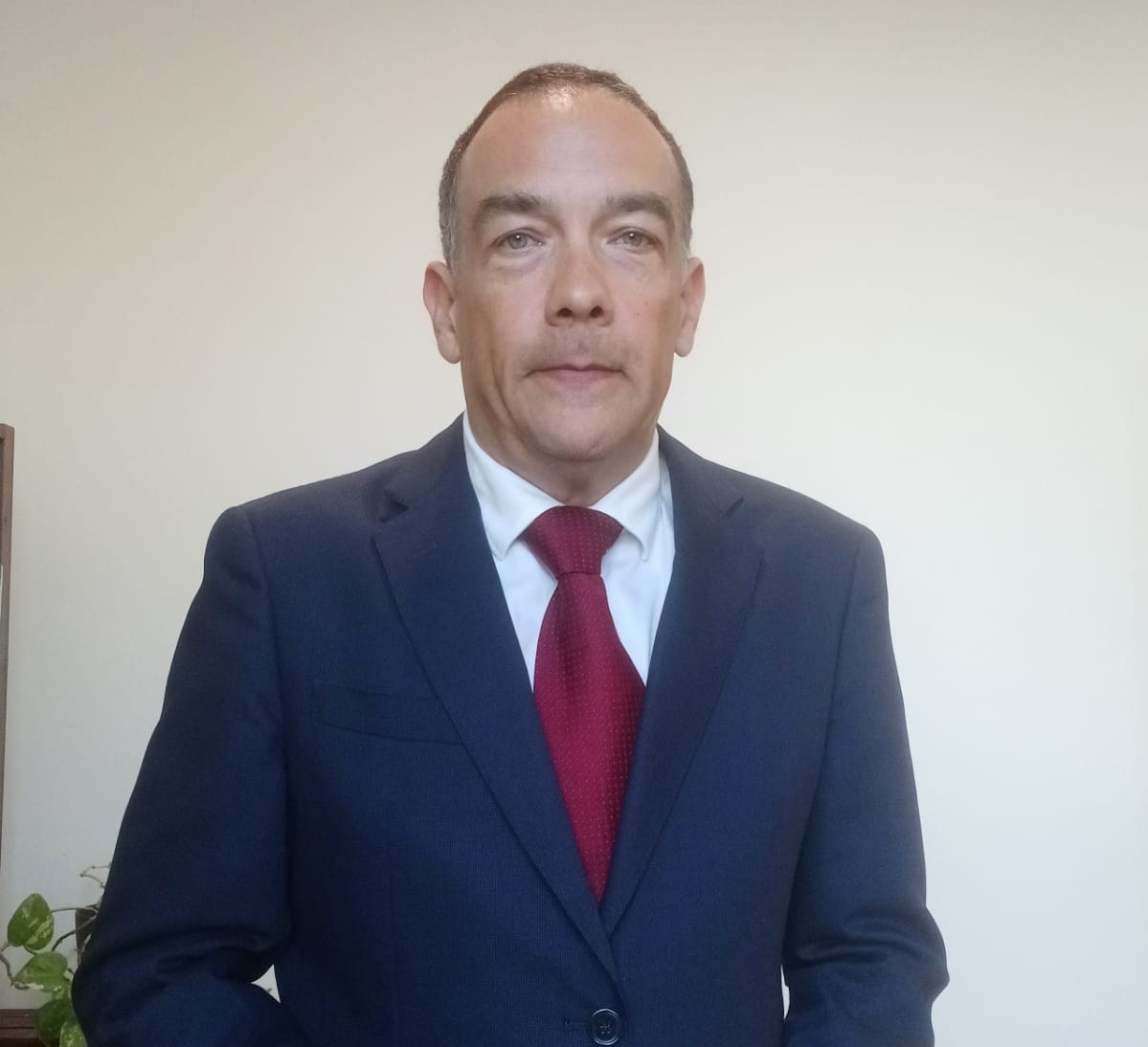
Andrzejak, Ralph Gregor, Department of Information and Communication Technologies, Universitat Pompeu Fabra, Barcelona, Spain
Talk Title: TBA
more | less
Dimitris Kugiumtzis is Professor on Computational Statistics – Time Series at the Department of Electrical and Computer Engineering (ECE). He was Associate Professor at ECE (2013-2017), Assistant and Associate Professor at the Department of Mathematical, Physical and Computational Sciences (2001-2013), Lecture B at the Department of Statistics, University of Glasgow (2000-2001), guest scientist (PostDoc) at the Max-Planck-Institute for Physics of Complex Systems in Dresden (1998-1999) and associate researcher at the State Center for Epilepsy in Norway (1997). He is director of the Informatics Lab ECE since 2019 and the Interdepartmental MSc on Biomedical Engineering (2021-2023). He supervised 8 PhD theses (other two in progress), over 40 MSc theses and 20 diploma theses. He has 95 peer-reviewed publications, 24 publications in international and 29 in national conference proceedings. He has h-index 27 and 2226 citations (source Web of Science, 11/5/2023).
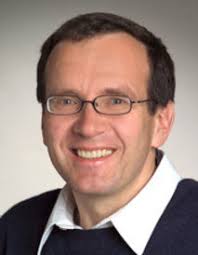
more | less
Dimitris Kugiumtzis is Professor on Computational Statistics – Time Series at the Department of Electrical and Computer Engineering (ECE). He was Associate Professor at ECE (2013-2017), Assistant and Associate Professor at the Department of Mathematical, Physical and Computational Sciences (2001-2013), Lecture B at the Department of Statistics, University of Glasgow (2000-2001), guest scientist (PostDoc) at the Max-Planck-Institute for Physics of Complex Systems in Dresden (1998-1999) and associate researcher at the State Center for Epilepsy in Norway (1997). He is director of the Informatics Lab ECE since 2019 and the Interdepartmental MSc on Biomedical Engineering (2021-2023). He supervised 8 PhD theses (other two in progress), over 40 MSc theses and 20 diploma theses. He has 95 peer-reviewed publications, 24 publications in international and 29 in national conference proceedings. He has h-index 27 and 2226 citations (source Web of Science, 11/5/2023).
Bodenschatz, Eberhard, Max Planck Institute for Dynamics and Self-Organization, Göttingen, Germany
Talk Title: TBA
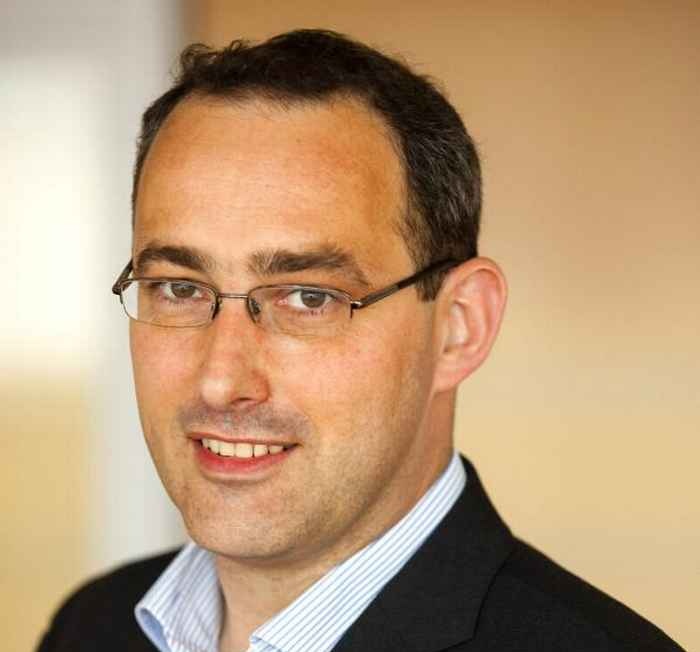
Diks, Cees G. H., Faculty of Economics and Business, University of Amsterdam, Amsterdam, The Netherlands
Talk Title: TBA
more | less
Dimitris Kugiumtzis is Professor on Computational Statistics – Time Series at the Department of Electrical and Computer Engineering (ECE). He was Associate Professor at ECE (2013-2017), Assistant and Associate Professor at the Department of Mathematical, Physical and Computational Sciences (2001-2013), Lecture B at the Department of Statistics, University of Glasgow (2000-2001), guest scientist (PostDoc) at the Max-Planck-Institute for Physics of Complex Systems in Dresden (1998-1999) and associate researcher at the State Center for Epilepsy in Norway (1997). He is director of the Informatics Lab ECE since 2019 and the Interdepartmental MSc on Biomedical Engineering (2021-2023). He supervised 8 PhD theses (other two in progress), over 40 MSc theses and 20 diploma theses. He has 95 peer-reviewed publications, 24 publications in international and 29 in national conference proceedings. He has h-index 27 and 2226 citations (source Web of Science, 11/5/2023).
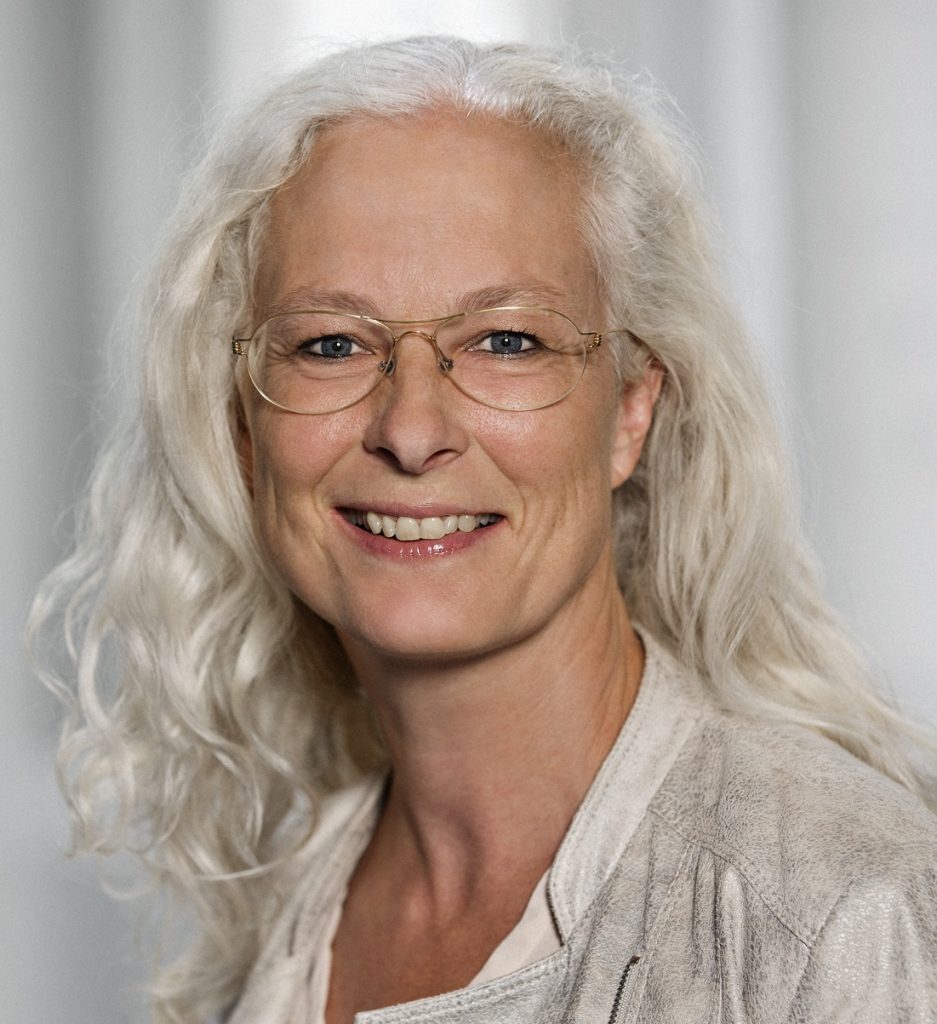
Ditlevsen, Susanne, Department of Mathematical Sciences, University of Copenhagen, Copenhagen, Denmark
Talk Title: TBA
more | less
Dimitris Kugiumtzis is Professor on Computational Statistics – Time Series at the Department of Electrical and Computer Engineering (ECE). He was Associate Professor at ECE (2013-2017), Assistant and Associate Professor at the Department of Mathematical, Physical and Computational Sciences (2001-2013), Lecture B at the Department of Statistics, University of Glasgow (2000-2001), guest scientist (PostDoc) at the Max-Planck-Institute for Physics of Complex Systems in Dresden (1998-1999) and associate researcher at the State Center for Epilepsy in Norway (1997). He is director of the Informatics Lab ECE since 2019 and the Interdepartmental MSc on Biomedical Engineering (2021-2023). He supervised 8 PhD theses (other two in progress), over 40 MSc theses and 20 diploma theses. He has 95 peer-reviewed publications, 24 publications in international and 29 in national conference proceedings. He has h-index 27 and 2226 citations (source Web of Science, 11/5/2023).
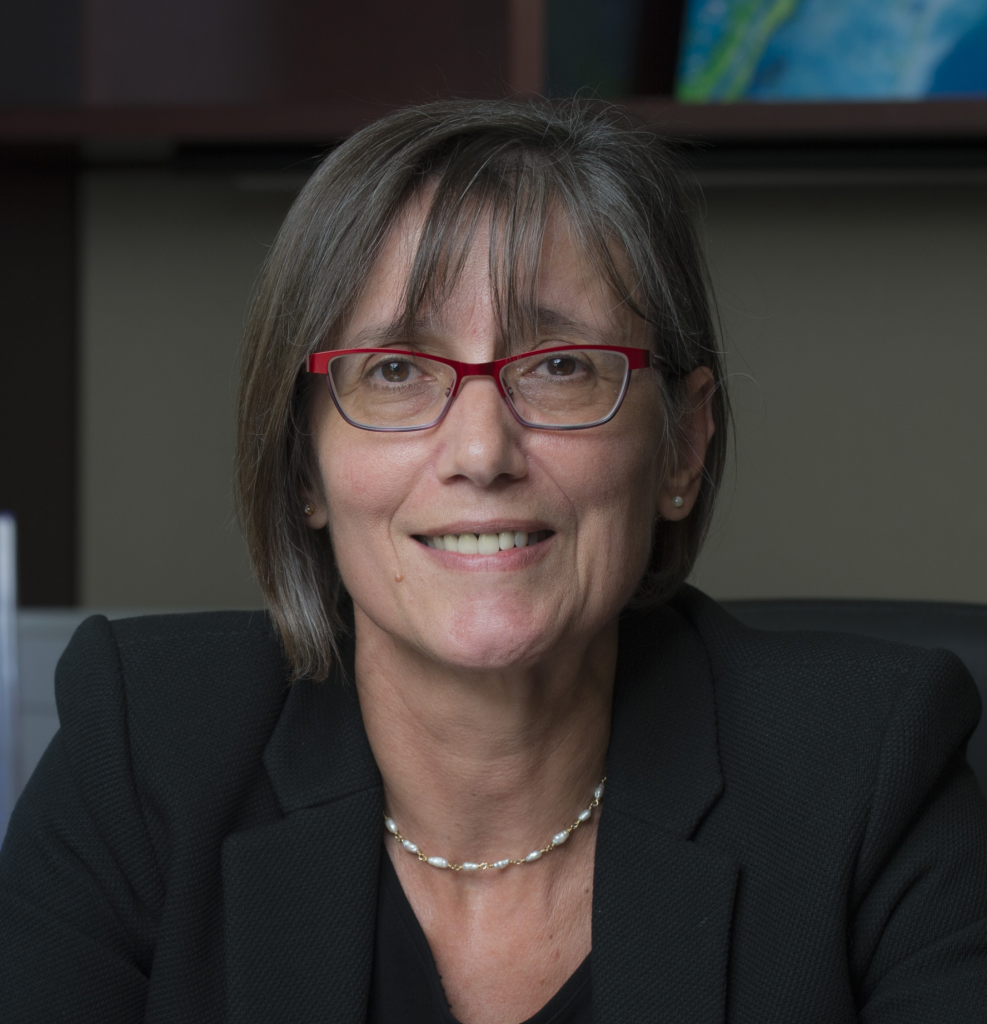
Foufoula-Georgiou, Efi, Departments of Civil and Environmental Engineering
and Earth System Science, University of California, Irvine (UCI), US
Talk Title: TBA
more | less
Dimitris Kugiumtzis is Professor on Computational Statistics – Time Series at the Department of Electrical and Computer Engineering (ECE). He was Associate Professor at ECE (2013-2017), Assistant and Associate Professor at the Department of Mathematical, Physical and Computational Sciences (2001-2013), Lecture B at the Department of Statistics, University of Glasgow (2000-2001), guest scientist (PostDoc) at the Max-Planck-Institute for Physics of Complex Systems in Dresden (1998-1999) and associate researcher at the State Center for Epilepsy in Norway (1997). He is director of the Informatics Lab ECE since 2019 and the Interdepartmental MSc on Biomedical Engineering (2021-2023). He supervised 8 PhD theses (other two in progress), over 40 MSc theses and 20 diploma theses. He has 95 peer-reviewed publications, 24 publications in international and 29 in national conference proceedings. He has h-index 27 and 2226 citations (source Web of Science, 11/5/2023).
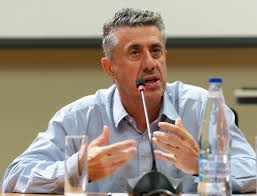
Karagiannidis, George, School of Electrical and Computer Engineering, Aristotle University of Thessaloniki, Thessaloniki, Greece
Talk Title: TBA
more | less
short bio …
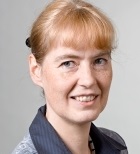
Krischer, Katharina, TUM School of Natural Sciences, Technical University of Munich, Munich, Germany
Talk Title: TBA
more | less
short bio …
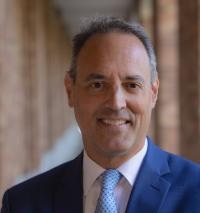
Kutz, J. Nathan, Department of Applied Mathematics, University of Washington, Washington, USA
Talk Title: TBA
more | less
short bio …
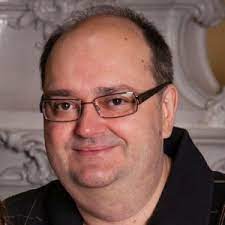
Paluš, Milan, Institute of Computer Science, Academy of Sciences of the Czech Republic, Prague, Czechia
Talk Title: TBA
more | less
short bio …
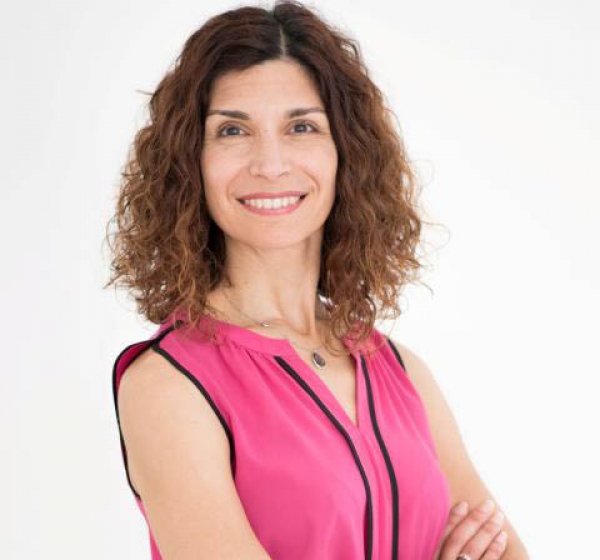
Poirazi, Panagiota, Institute of Molecular Biology and Biotechnology, The Foundation for Research and Technology – Hellas (FORTH), Heraklion, Greece
Talk Title: TBA
more | less
short bio …
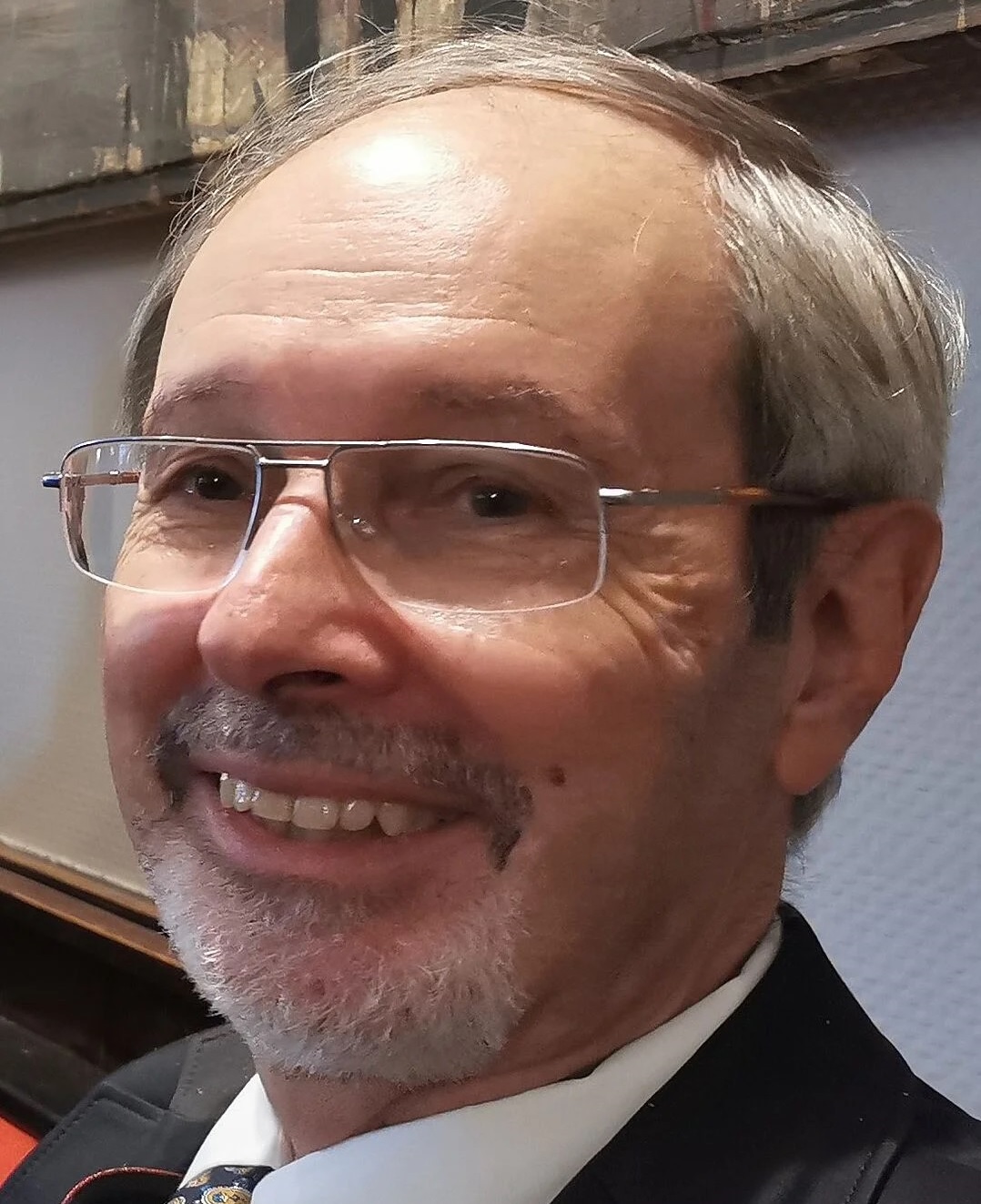
Schöll, Eckehard, Institute for Theoretical Physics, Technische Universität Berlin, Berlin, Germany
Talk Title: TBA
more | less
short bio …
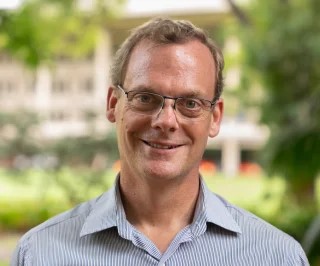
Small, Michael, School of Physics, Maths and Computing, University of Western Australia, Perth, Australia
Talk Title: TBA
more | less
short bio …